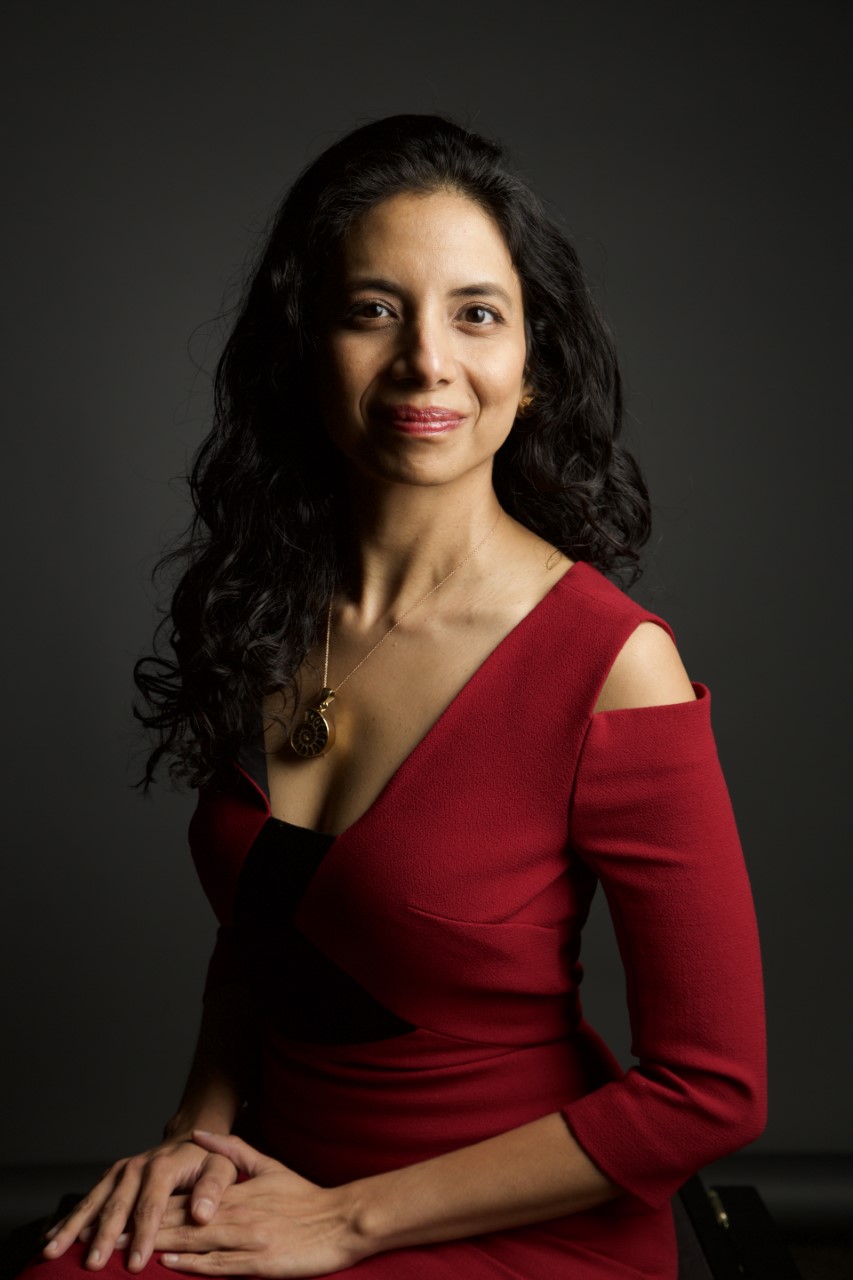
distinguished lecture series presents
Anima Anandkumar
Caltech
Research Area
Large-scale machine learning, non-convex optimization and high-dimensional statistics
Visit
Wednesday, April 13th, 2022 to Thursday, April 14th, 2022
Location
MS 6627 and Zoom https://ucla.zoom.us/j/9264073849